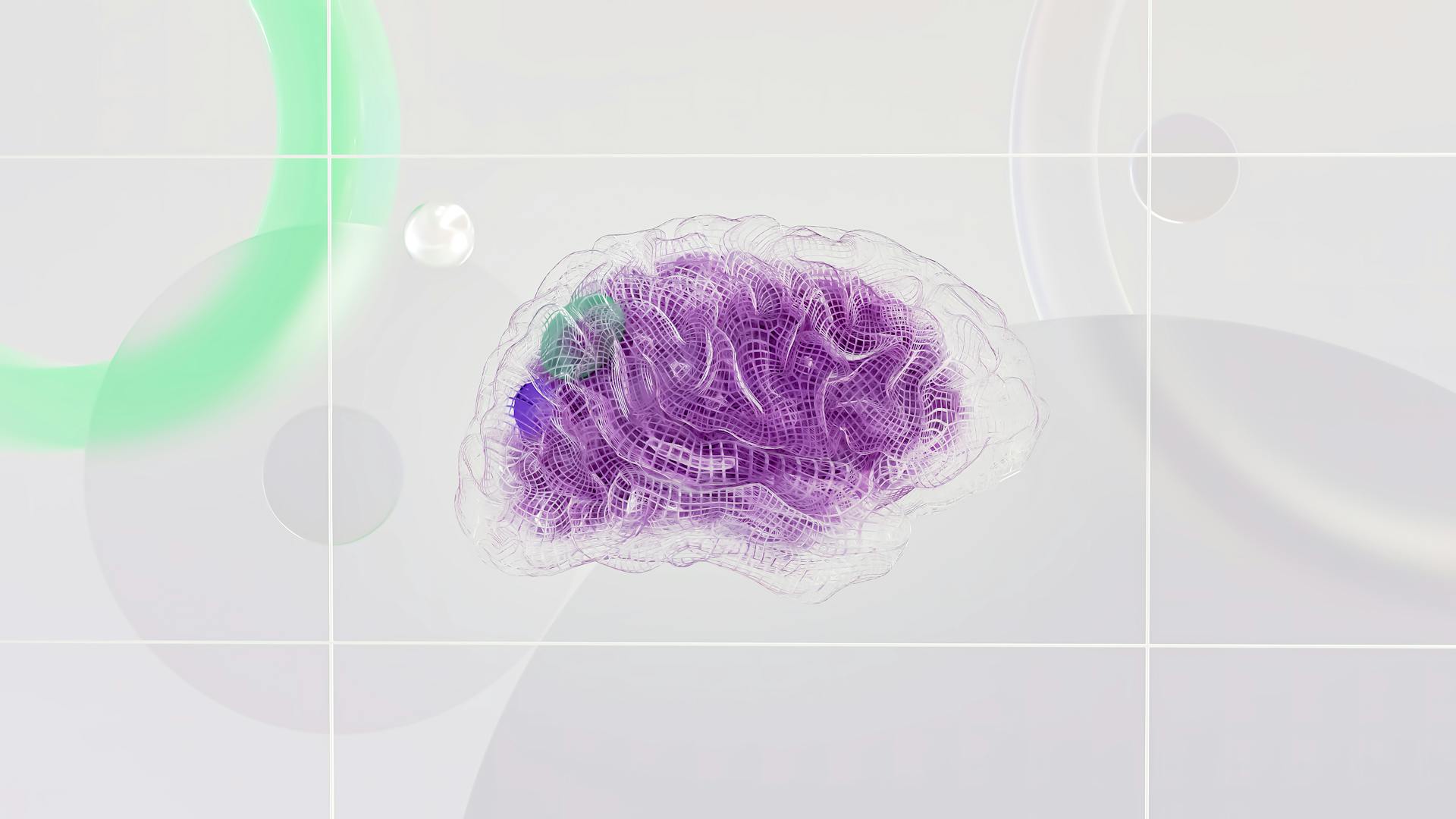
Machine learning is revolutionizing the way we approach agriculture, making it more efficient and sustainable. By analyzing vast amounts of data, machine learning algorithms can predict crop yields, detect early signs of disease, and optimize irrigation systems.
Farmers can use machine learning to identify the most suitable crops for their soil and climate, reducing waste and increasing productivity. For example, a study found that using machine learning to optimize crop selection increased yields by 20%.
Precision farming is another area where machine learning is making a significant impact. By using drones and sensors to collect data, farmers can create detailed maps of their fields, identifying areas that need more attention. This approach has been shown to reduce chemical use by 30% and increase yields by 15%.
Machine learning can also help farmers predict and prepare for extreme weather events, such as droughts and floods. By analyzing historical weather data and real-time satellite imagery, machine learning algorithms can provide early warnings and recommendations for farmers to take action.
What is Machine Learning in Agriculture?
Machine learning in agriculture is a game-changer. It uses algorithms and data to give machines the ability to learn like humans, automating processes and improving efficiency.
Many applications for machine learning are used in agriculture, including image and speech recognition, natural language processing, and predictive modeling. These solutions allow farmers to make the most of their data, improve decision-making, and provide high-quality services.
Machine learning allows businesses and industries to improve decision-making and provide high-quality services. This is especially true in agriculture, where data-driven insights can help farmers optimize crop yields, reduce waste, and increase profitability.
Consider reading: Agriculture Machine with Name
Benefits and Applications
Machine learning in agriculture offers numerous benefits that can transform the way farmers work. It helps reduce environmental risks by optimizing irrigation schedules, fertilizer use, and pesticide use.
Machine learning automates tasks like field mapping, crop health monitoring, and fertilizer application, saving farmers time and money. This can also reduce their need for hired labor.
One of the key advantages of machine learning in agriculture is its ability to improve yield by optimizing resources. This can lead to increased crop yields, improving food security and reducing hunger.
Machine learning also helps farmers save on vital resources such as water, fertilizers, and pesticides, increasing profitability and making agriculture more sustainable in the long run.
Here are some of the specific benefits of machine learning in agriculture:
- Reducing environmental risks by optimizing irrigation schedules, fertilizer use, and pesticide use
- Save time and labor by automating field mapping, crop health monitoring, and fertilizer application
- Improving yield by optimizing resources
- Cost reduction by saving on vital resources such as water, fertilizers, and pesticides
- Improved decision-making by providing farmers with better insights
- Increased safety by avoiding dangerous tasks
- Providing personalized advice for planting, watering, and fertilizing
- Building resilience to climate change by adapting practices to weather patterns
- Biodiversity Conservation by managing land to preserve ecosystem services
- Improving food quality by producing safer and better-quality food
13 Use Cases
Machine learning is revolutionizing the way we approach agriculture, making it more efficient, sustainable, and productive. One of the most significant advantages of machine learning in agriculture is its ability to predict crop yields, which can help farmers plan and manage their harvests more effectively.
Here are 13 use cases for machine learning in agriculture, each with its own unique benefits and applications:
Crop management is a crucial aspect of agriculture, and machine learning can help with tasks such as:
- Yield prediction
- Disease detection
- Weed detection
- Crop recognition
- Grading by quality
- Selective breeding
Water management is also a critical component of agriculture, and machine learning can help with tasks such as:
- Irrigation
- Soil water monitoring
- Leak detection
- Weather monitoring
- Weather prediction
- Water usage prediction
Soil management is another area where machine learning can have a significant impact, helping with tasks such as:
- Pesticides and fertilizers
- Fertility prediction
- Soil sensitivity
- Moisture prediction
- Organic carbon prediction
- Insect detection
Livestock management is also an area where machine learning can be applied, helping with tasks such as:
- Animal welfare monitoring
- Precision livestock farming
- Monitoring production quality
- Monitoring living conditions
- Grazing control
- Disease detection
Machine learning models can also be used to analyze data and make predictions in agriculture, such as predicting crop yields, detecting pests and diseases, and optimizing irrigation.
Technologies and Approaches
Machine learning in agriculture is transforming traditional farming methods by simplifying and improving agricultural processes. AI subsets and ML technologies, such as deep learning, computer vision, and predictive analytics, can help farmers make informed decisions.
Regression algorithms can be used to predict crop yields by considering factors such as historical data, weather patterns, soil composition, and management practices. This information can empower farmers to optimize resource allocation and make informed decisions.
Supervised machine learning models can be divided into regression and classification categories, depending on the type of problem being addressed. Regression algorithms are used for predictive modeling, while classification algorithms involve identifying categories of new observations based on independent variables.
Unsupervised machine learning involves algorithms that identify patterns based on similarities and differences in information. Farmers can apply these algorithms in crop management to group plants based on different characteristics or according to pre-analyzed conditions under which particular crops are going to thrive.
Discover more: Itil Change Management Implementation Guide
Technologies
Machine learning models can be divided into supervised and unsupervised categories depending on the type of data used for their training algorithms.
Supervised models can be further divided into regression and classification problems. Regression algorithms are used for predictive modeling to determine and analyze the relation between one dependent variable and a series of other variables.
For instance, applying regression algorithms, farmers and enterprises can predict yield based on historical dataset, weather and management data, soil information, and other factors. Selective breeding can also be approached using regression algorithms to identify crops with desirable traits such as resistance to diseases and other.
Classification algorithms involve identifying the categories of new observations based on independent variables. In smart farming using machine learning, for example, they can be used to classify and filter crops.
Unsupervised machine learning involves algorithms that identify patterns based on similarities and differences in information. Farmers and enterprises can apply these algorithms in crop management to group plants based on different characteristics or according to pre-analyzed conditions under which particular crops are going to thrive.
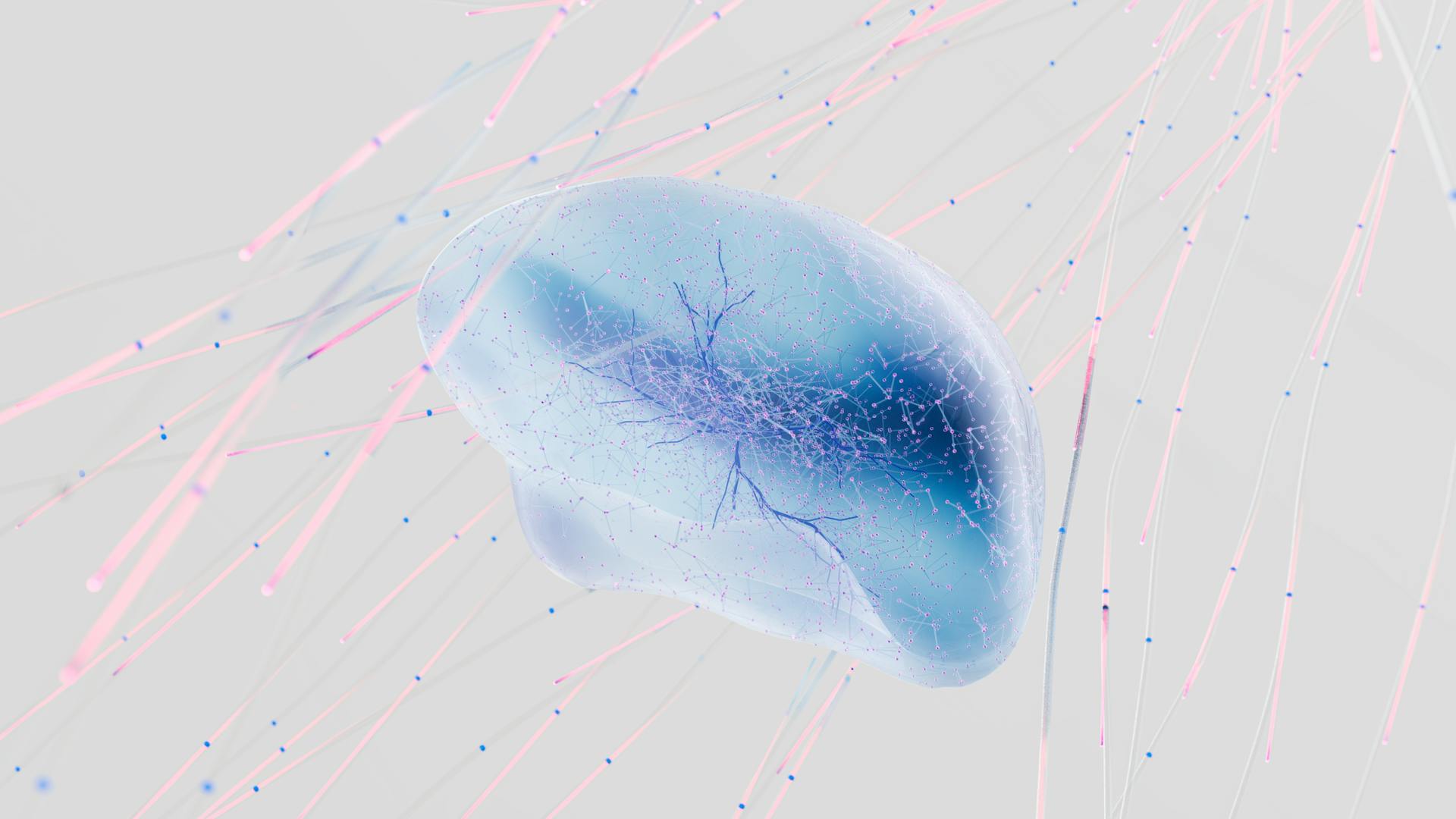
Regression models can predict crop yields, the price of agricultural commodities, and the demand for agricultural products. Bayesian models can provide accurate crop yield predictions by using data on weather, soil conditions, and other factors.
Artificial neural networks are well-suited for agricultural applications because they can learn to identify patterns in data that are too complex for humans to discern. For example, artificial neural networks can be used to develop new strains of crops that are more resistant to pests or diseases.
Approaches
Machine learning models in agriculture can be divided into supervised and unsupervised categories, depending on the type of data used for their training algorithms.
Supervised models excel at predicting continuous values, such as forecasting crop yields by considering factors like historical data, weather patterns, soil composition, and management practices.
Regression algorithms are used for predictive modeling, allowing farmers to predict yield based on historical dataset, weather, and management data, soil information, and other factors.
Classification algorithms, another cornerstone of supervised learning, can be employed to classify crop varieties, detect plant diseases, or predict pest outbreaks.
Unsupervised models uncover hidden patterns within data without prior labeling, allowing farmers to group plants based on similar characteristics, genetic makeup, or environmental preferences.
Clustering algorithms can be used to identify which plants are more likely to thrive in certain conditions and develop targeted interventions.
Artificial neural networks can be used to develop new strains of crops that are more resistant to pests or diseases by learning to identify patterns in data that are too complex for humans to discern.
Bayesian models can provide accurate crop yield predictions by using data on weather, soil conditions, and other factors, helping farmers decide what crops to plant, how much fertilizer to use, and when to harvest.
Machine learning applications in agriculture are transforming traditional farming methods, enabling precision-based approaches that optimize resource use and predict yields.
Discover more: Can a Ac Motor Be Used as a Generator
Data
Machine learning can efficiently process and analyze lots of complex data that can be used for making informed decisions and building strategies.
Farmers and agricultural businesses generate an immense amount of data, which can be hard to manage without the assistance of ML technologies.
Predictive analytics refers to data analysis methods that are focused on forecasting outcomes using historical data together with machine learning, data mining, and statistical modeling to improve decision-making and operational efficiencies and reduce risks.
In agriculture, predictive analytics can help forecast demand, irrigation requirements, crop yield, and weather conditions.
Recurrent neural networks (RNNs) reveal insights from temporal data like weather records, crop growth curves, and commodity prices.
Machine learning tools can analyze environmental data from multiple locations and predict whether some insect swarm would affect your farm negatively in the first place.
The ability to work with tremendous amounts of data and then manage it is the core benefit of modern machine learning tools.
Data management is one more aspect that greatly benefits from machine learning in agriculture, allowing farmers to make informed decisions about when to plant, how to care for their crops, and what prices to charge for their produce.
A fresh viewpoint: Baler Machine Agriculture
Predictive Analytics
Predictive analytics is a powerful tool in agriculture, helping farmers make informed decisions about crop yields, demand, and resource allocation.
Predictive analytics can forecast demand, irrigation requirements, crop yield, and weather conditions, allowing farmers to make informed decisions on when and where to plant crops.
By analyzing past data patterns, predictive analytics can provide insights that help farmers optimize irrigation and fertilizer usage, leading to more efficient and sustainable farming practices.
Farmers can rely on predictive analytics to make decisions on crop selection, planting, and harvesting, reducing the risk of crop failure and increasing productivity.
Predictive analytics can also identify early warning signs of crop pests and diseases, enabling farmers to take preventive measures and avoid potential damage.
With predictive analytics, farmers can make data-driven decisions, reducing the reliance on intuition and guesswork.
Farmers can use predictive analytics to predict crop yields, the price of agricultural commodities, and the demand for agricultural products, helping them stay competitive in the market.
Predictive analytics can be used to study the impact of new technologies on agriculture, allowing farmers to evaluate the efficiency of different agricultural production planning systems.
Farmers can use predictive analytics to optimize resource allocation, make informed decisions about crop selection and planting, and reduce the risk of crop failure.
Predictive analytics can help farmers make decisions on how to efficiently distribute resources, encouraging sustainability and reducing waste.
Farmers can rely on predictive analytics to make informed decisions about crop yields, demand, and resource allocation, leading to more efficient and sustainable farming practices.
Predictive analytics can provide accurate crop yield predictions by using data on weather, soil conditions, and other factors, empowering farmers to make informed decisions.
Farmers can use predictive analytics to decide what crops to plant, how much fertilizer to use, and when to harvest, leading to increased productivity and reduced waste.
Predictive analytics can help farmers make decisions on crop selection, planting, and harvesting, reducing the risk of crop failure and increasing productivity.
By using predictive analytics, farmers can optimize their farming practices, leading to increased productivity, reduced waste, and improved sustainability.
Check this out: Automated Farming Equipment
Computer Vision and Image Processing
Computer vision is a machine learning field that collects and analyzes data from digital images, videos, and other visual inputs trying to mimic the way human eyes work.
It can simultaneously process and gather unlimited amounts of information, unlike humans, enabling object identification, measuring, movement tracking, and other processes.
Computer vision has a wide range of applications in smart agriculture, from yield prediction and crop monitoring to precision irrigation and automated farm machinery.
Monitoring crop health is one of the most important applications of computer vision in agriculture, allowing farmers to detect early signs of disease or stress that may not be visible to the naked eye.
Deep convolutional neural networks (CNNs) accurately analyze imagery for plant identification, disease spotting, and weed mapping tasks.
Machine learning can also automate the weeding process, such as an ML-based robot that targets individual unwanted plants and removes broad-leaved weeds using pattern recognition.
Detecting locations of unwanted weed species amidst crops using pixel-wise semantic segmentation models on UAV imagery can guide selective treatment.
Environmental and Sustainability
Machine learning in agriculture can help farmers optimize water usage, fertilizer and herbicide application, and irrigation schedules to reduce wastage and environmental harm. This can lead to minimized environmental impacts on farms.
Farmers can use machine learning algorithms to analyze data from various sources, including weather patterns, soil composition, and crop yields, to identify areas where sustainability can be improved. By leveraging this data-driven approach, farmers can optimize their operations and reduce waste.
Precision agriculture, enabled by machine learning, can optimize resource inputs, reduce waste, and minimize environmental harm. This approach uses advanced sensors, drones, and satellite imagery to precisely monitor crop growth, soil conditions, and water usage.
Machine learning can also help farmers adopt regenerative agriculture practices, such as rebuilding soil health, enhancing biodiversity, and sequestering carbon. By embracing these sustainable practices, farmers can improve the long-term productivity of their land and contribute to mitigating the effects of climate change.
Farmers must carefully consider the potential environmental impact of machine learning in farming and prioritize sustainable practices. A comprehensive life cycle assessment of these systems is essential to ensure that they do not exacerbate existing problems.
By analyzing and optimizing water and soil resources, farmers can minimize negative environmental consequences of pesticides and crop disease treatments. This can lead to improved supply chain logistics and a more sustainable agricultural sector.
Worker Safety
Worker safety is a top concern in agriculture, and machine learning can play a significant role in improving it. Machine learning helps farmers avoid hazardous tasks such as working with pesticides.
Automating tasks like working with heavy machinery can greatly reduce the risk of accidents and injuries on farms. Machine learning systems can eliminate the need for farmers to put their lives in danger by taking over these tasks.
Certain activities in agriculture are potentially hazardous, and machine learning tools can help control machines in an automatic mode. This capability of machine learning technology can be capitalized on to automate farms through a data-driven approach.
Precision Agriculture
Precision agriculture is a trendy term, and machine learning is at its core. This approach involves tailoring farming practices to the unique needs of different sections of a field.
Machine learning algorithms process data from IoT devices, drones, and sensors to create detailed maps of soil characteristics, moisture levels, and crop health. These insights enable farmers to apply fertilizer, irrigation, and pesticides precisely where they are needed, reducing environmental impact and optimizing resource use.
Farmers can use machine learning to analyze crop health and optimize resource use. Precision agriculture can also help farmers make educated guesses about weather conditions and adjust irrigation accordingly.
Big data and machine learning tools become essential for making advanced predictions in the sphere of agriculture. This approach enables farmers to optimize the use of resources through the analysis of weather patterns.
Machine learning in agriculture can help farmers create detailed maps of soil characteristics, moisture levels, and crop health. This information can be used to tailor farming practices to the unique needs of different sections of a field.
Curious to learn more? Check out: Modern Farming Equipment
Livestock and Animal Health
Machine learning is helping farmers detect animal diseases early on by analyzing images and videos of animals with computer vision. This allows for the early identification of diseases and prevention of sickness spreading.
Farmers can use machine learning to monitor animals' health, daily activity levels, food intake, and other factors that impact production. This helps prevent losses among the livestock and ensures high yields without contamination.
By tracking animal movement, behavior, and health with computer vision, wearables, and anomaly detection algorithms, farmers can enable early disease diagnosis and maintain high yields. Machine learning in agriculture also helps with detecting diseases early on by reviewing the sporadic behavior of involved animals.
Livestock and Animal Health
Machine learning algorithms can detect animal diseases by continuously analyzing images and videos of animals with the help of computer vision. This allows for the early identification of diseases and prevention of sickness spreading.
Diseases in animals can have devastating impacts, such as the CJD outbreak in the UK during the year 2000. Machine learning technology helps detect such problems early on by reviewing the sporadic behavior of involved animals.
Livestock management requires timely detection of diseases and immediate action to prevent their spreading. ML technologies can help monitor animals' health, daily activity levels, food intake, and other factors that impact production.
Machine learning can analyze livestock feeding habits to disclose animals' nutrition patterns and abnormalities. This helps prevent overgrazing by planning when and where animals should graze based on factors like livestock density, land cover type, and weather.
Livestock monitoring is crucial for early disease diagnosis, and machine learning enables this by tracking animal movement, behavior, and health with computer vision, wearables, and anomaly detection algorithms.
Feeding is an essential component in raising animals, and machine learning can fully automate feeding with the help of sensors and dispenser systems. This prevents long-term problems with animal health and farm yields.
Breeding Assistance
Machine learning algorithms can help you select the most positive traits in your livestock, going beyond algorithmic predictions to offer more advanced predictions.
With the help of machine learning tools, you can analyze the lineages of several animals to make predictions on the manifestation of particular traits, offering accuracy that no other tools can match.
A single hybrid development cycle in plant breeding can take scientists seven or eight years without the aid of ML-based technology, but ML algorithms can help optimize the identification of biotech traits needed to increase yields.
Machine learning helps scientists make predictions regarding which gene combinations will lead to desirable traits in new plants, providing an excellent starting point for developing hardier plant species.
By evaluating masses of data on plant performance in various conditions over time, ML algorithms can help scientists reduce resistance buildup and improve the longevity of biotech traits.
Frequently Asked Questions
Can AI take over agriculture?
AI in agriculture is not about replacing farmers, but about augmenting their work with precision and efficiency. By leveraging AI, farmers can stay competitive and improve their operations, but it's a tool to be explored and integrated, not a replacement.
Sources
- https://www.itransition.com/machine-learning/agriculture
- https://www.effectivesoft.com/blog/machine-learning-in-agriculture.html
- https://keenethics.com/blog/machine-learning-in-agriculture-importance-and-key-use-cases
- https://saiwa.ai/blog/machine-learning-in-agriculture/
- https://objectcomputing.com/industries/agriculture
Featured Images: pexels.com